How to Master Probability Density Functions
A Probability Density Function (\(PDF\)) is a cornerstone concept in statistics, particularly when dealing with continuous random variables. Here is a step-by-step guide to understanding Probability Density Functions.
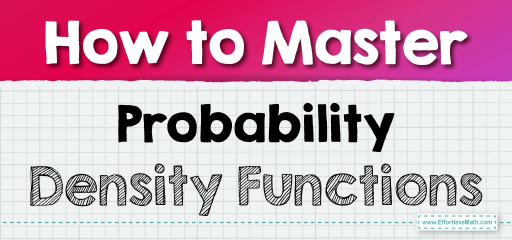
Step-by-step Guide to Master Probability Density Functions
Here is a step-by-step guide to mastering probability density functions:
Step 1: Understand Continuous Random Variables
- A continuous random variable can take an infinite number of possible values. For example, the exact height of adult males in a city is continuous—it could be \(170.1234 \ cm\), \(170.1235 \ cm\), etc.
Step 2: Define the Probability Density Function (\(PDF\))
- The \(PDF\), denoted usually as \(f(x)\), is a function that describes the relative likelihood of a continuous random variable to take on a certain value. Unlike discrete random variables, continuous variables require a function to represent probabilities over intervals.
Step 3: Properties of the \(PDF\)
- Non-negativity: The \(PDF\) must be non-negative \(f(x)≥0\) for all \(x\), since probabilities cannot be negative.
- Area equals \(1\): The area under the entire \(PDF\) curve equals \(1\), representing the fact that the random variable will take on a value within the range.
- Interval Probabilities: The probability that the random variable \(X\) falls within an interval \([a,b]\) is the area under the \(PDF\) curve from \(a\) to \(b\), calculated by the integral \({∫_a}^{b}f(x) \ dx\).
Step 4: Computing Interval Probabilities
- To compute the probability of the variable falling within a specific range, integrate the \(PDF\) over that range. This area under the curve represents the probability for that interval.
Step 5: Probability of Exact Values
- For continuous random variables, the probability of taking any exact value is zero. This is due to the infinite number of possible values, so the probability of \(X\) equaling a specific \(x\) is \(P(X=x)=0\).
Step 6: Mean and Variance from the \(PDF\)
- The mean (or expected value) of a continuous random variable can be found by integrating \(x⋅f(x)\) over all possible values of \(x\).
- The variance can be computed by integrating \((x−μ)^2⋅f(x)\) over all \(x\), where \(μ\) is the mean.
Step 7: Graphing the \(PDF\)
- Visualizing the \(PDF\) as a graph is crucial for understanding the distribution of the random variable. The height of the function at any point can be interpreted as the density of probability.
Step 8: Examples of \(PDF\)s
- Familiarize yourself with common \(PDF\)s, such as the normal distribution, which is bell-shaped, or the uniform distribution, where the probability is evenly spread across an interval.
Step 9: Practical Applications
- Use the \(PDF\) to solve real-world problems, such as determining the probability that a measured temperature falls within a certain range on a given day.
Step 10: Advanced Concepts
- Once you are comfortable with the basics, explore more complex topics like joint \(PDF\)s for multiple random variables and transformation of random variables.
Understanding \(PDF\)s is essential for analyzing and making predictions about data that vary continuously, which is a fundamental aspect of statistical analysis and many scientific disciplines.
Related to This Article
More math articles
- How to Evaluate Trigonometric Function? (+FREE Worksheet!)
- The Ultimate 6th Grade RICAS Math Course (+FREE Worksheets)
- HiSET Math Practice Test Questions
- ACT Calculators: Exреrt Tiрѕ
- Visualizing the Magic: How to Multiply Fractions Using Models
- Full-Length AFOQT Math Practice Test-Answers and Explanations
- How to Find Magnitude of Vectors?
- How to Draw Geometric Shapes with given condition?
- TSI Math FREE Sample Practice Questions
- 3rd Grade STAAR Math Practice Test Questions
What people say about "How to Master Probability Density Functions - Effortless Math: We Help Students Learn to LOVE Mathematics"?
No one replied yet.