How to Demystifying the Bell Curve: A Comprehensive Guide to Understanding Normal Distribution
Understanding the normal distribution, often referred to as the bell curve due to its shape, is fundamental in statistics. Here's a step-by-step guide to help you understand this concept:
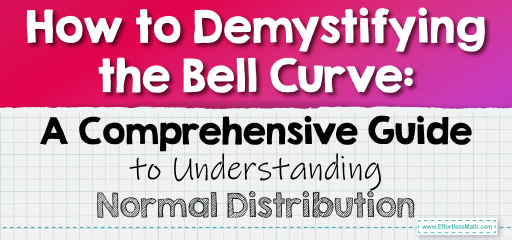
Step-by-step Guide to Demystifying Normal Distribution
Step 1: Conceptual Understanding of Normal Distribution
- Definition: The normal distribution is a probability distribution that is symmetric about the mean, showing that data near the mean are more frequent in occurrence than data far from the mean.
- Shape: It has a bell-shaped curve, with the peak at the mean, and it is symmetric around this mean.
- Properties:
- The total area under the curve is \(1\).
- It is described by two parameters: the mean (\(μ\)) and the standard deviation (\(σ\)).
- About \(68%\) of the data falls within one standard deviation of the mean, \(95%\) within two standard deviations, and \(99.7%\) within three standard deviations (this is known as the \(68-95-99.7\) rule or the empirical rule).
Step 2: Mathematical Foundation
- Equation: The normal distribution can be mathematically represented by the formula of the probability density function (PDF): \(f(x)=\)\(\frac{1}{σ\sqrt{2π}}e^{−\frac{1}{2}(\frac{x−μ}{σ})^2}\)
- Curve Characteristics: The curve is higher and narrower when the standard deviation is small, and flatter and wider when the standard deviation is large.
Step 3: Graphical Representation
- Plotting the Curve: To visualize the normal distribution, you can plot the probability density function using the mean and standard deviation of your data.
- Symmetry: The left and right halves of the curve are mirror images.
Step 4: Real-World Examples
- Understanding Examples: Many natural phenomena are normally distributed, such as heights or IQ scores in a population. This makes normal distribution extremely useful in statistics for making predictions and inferences about a larger population from sample data.
Step 5: Applications in Statistics
- Standard Normal Distribution: This is a special case of the normal distribution where the mean is \(0\) and the standard deviation is \(1\). It’s used for z-scores in statistics.
- Central Limit Theorem: This theorem states that the distribution of sample means approximates a normal distribution as the sample size gets larger, regardless of the shape of the population distribution.
Step 6: Practical Exercise
- Gather Data: Collect or find a dataset.
- Calculate Mean and Standard Deviation: Use statistical methods to find these values for your dataset.
- Plot the Distribution: Use software or graphing techniques to plot the normal distribution curve with your data.
- Compare and Analyze: See how closely your data follows a normal distribution. Use this to make predictions or understand data variability.
Step 7: Common Misconceptions
- Not All Data is Normally Distributed: It’s a common mistake to assume all data sets follow a normal distribution. Only when the data is symmetrically distributed around the mean is it possibly normal.
- The Role of Outliers: Be mindful of outliers as they can significantly affect the mean and standard deviation, leading to a misinterpretation of the distribution.
Understanding the normal distribution is crucial for statistical analysis and hypothesis testing, as it provides a framework for understanding randomness and variability in data.
Examples:
Example 1:
Determine whether the following data set is likely to be from a normal distribution.
\(55, 60, 65, 70, 75, 80, 85, 90, 95, 100\)
Solution:
- Calculate Mean and Median: For a normal distribution, the mean and median are approximately equal.
- Mean = \(\frac{(55 + 60 + 65 + 70 + 75 + 80 + 85 + 90 + 95 + 100)}{10} = 77.5\)
- Median = \(\frac{(75+ 80)}{2}= 77.5\)
- Assessment: Since the mean and median are approximately equal, this data set can be from a normal distribution.
Example 2:
Assess if the following data set is from a normal distribution.
\(5,10,20,30,40,50,60,70\)
Solution:
- Mean Calculation: \(\frac{(5 + 10 + 20 + 30 + 40 + 50 + 60 + 70 )}{8} = 35.6\)
- Median Calculation: \(\frac{(30 + 40)}{2} = 35\)
- Assessment: Since the mean and median are not approximately equal, this data set is likely not from a normal distribution.
Related to This Article
More math articles
- Top 10 7th Grade STAAR Math Practice Questions
- Top 10 Tips to ACE the ASVAB Math
- Block by Block: How to Complete Decimal Division Equations
- Top 10 Tips You MUST Know to Retake the SSAT Math
- Using Vertical and Horizontal Number Lines to Represent Integers
- How to Write a Quadratic Function from Its Vertex and Another Point
- How to Unlock the Secrets: “SIFT Math for Beginners” Solution Manual
- Best Laptops for Online Math Teaching
- 10 Most Common 8th Grade FSA Math Questions
- Top 10 Tips to Create an AFOQT Math Study Plan
What people say about "How to Demystifying the Bell Curve: A Comprehensive Guide to Understanding Normal Distribution - Effortless Math: We Help Students Learn to LOVE Mathematics"?
No one replied yet.