Balancing Probabilities: A Comprehensive Guide to the Expected Value of Random Variables
Understanding the expected value of a random variable is akin to pinpointing the center of gravity in physics—it gives you the balance point of a distribution of values. Here’s a step-by-step guide to grasping this concept.
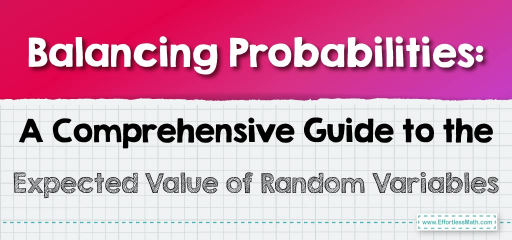
Step-by-step Guide to Grasping the Expected Value of Random Variables
Here is a step-by-step guide to grasping the expected value of random variables:
Step 1: Define Expected Value
- The expected value (\(EV\)) or expectation of a random variable is essentially a measure of the center or average of a probability distribution. It’s what you would expect as the outcome over a large number of trials.
Step 2: Expected Value for Discrete Random Variables
- For a discrete random variable \(X\), which takes on possible values \(x_1,x_2,…,x_n\) with corresponding probabilities \(p(x_1),p(x_2),…,p(x_n)\), the expected value is calculated using the formula:\(E[X]=∑_{i=1}^ {n}x_i⋅p(x_i)\)
- This is a sum of the value of each outcome times its probability.
Step 3: Expected Value for Continuous Random Variables
- For continuous random variables, the concept is similar, but instead of a sum, you use an integral because the variable can take on an infinite number of values within an interval. The expected value is: \(E[X]=∫_{−∞}^{∞}x⋅f(x) \ dx\)
- Here, \(f(x)\) is the probability density function, and \(x⋅f(x)\) gives a weighted value at each point, which you integrate over the entire range of possible values.
Step 4: Weighted Average Interpretation
- For both discrete and continuous cases, think of the expected value as a weighted average where each value is weighted by its likelihood of occurrence.
Step 5: Calculating the Expected Value
- For a discrete random variable, list out all possible outcomes and their probabilities. Multiply each outcome by its probability and sum all these products.
- For a continuous random variable, set up the integral of \(x⋅f(x)\) and calculate it over the range where the random variable exists.
Step 6: Center of Mass Analogy
- The “center of mass” analogy helps in understanding that the expected value is the balancing point of the distribution. If you could place the distribution on a fulcrum, it would balance at the expected value.
Step 7: Practical Examples
- In real-world terms, if you’re looking at the expected value of rolling a six-sided die, you multiply each side’s value (\(1\) through \(6\)) by the probability of rolling it (\(\frac{1}{6}\)), adding these together to get the expected value.
Step 8: Applications of Expected Value
- Expected value isn’t just an abstract concept; it’s used in insurance, finance, gambling, and other fields to determine fair prices, premiums, or to understand long-term gains or losses.
Step 9: Variability Around the Expected Value
- It’s important to note that the expected value is an average; individual outcomes may vary. The concept of variance and standard deviation builds on the expected value to measure the spread of the distribution.
Step 10: Misinterpretations to Avoid
- An expected value does not guarantee an outcome. For example, the expected value of a lottery ticket may be positive, but buying a ticket does not ensure a win.
By following these steps, you should have a solid understanding of expected values and their calculation for both discrete and continuous random variables, providing you with a deeper insight into the behavior of random processes.
Related to This Article
More math articles
- Line Segments
- Properties of the Horizontal Line
- How to Score 800 on SAT Math?
- Bеѕt Strategies to pass the Aссuрlасеr Test
- Top 10 ISEE Lower Level Prep Books (Our 2023 Favorite Picks)
- 3rd Grade NHSAS Math Worksheets: FREE & Printable
- Everything You Need to Know to Pass Algebra 1
- Top 10 Tips to Overcome SAT Math Anxiety
- The Ultimate Adults Algebra Refresher Course (+FREE Worksheets & Tests)
- 6th Grade PSSA Math Worksheets: FREE & Printable
What people say about "Balancing Probabilities: A Comprehensive Guide to the Expected Value of Random Variables - Effortless Math: We Help Students Learn to LOVE Mathematics"?
No one replied yet.